Breadcrumb
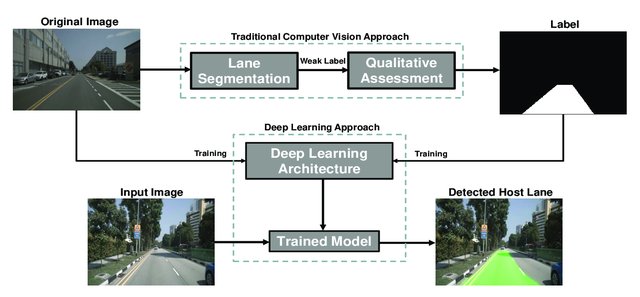
A Deep Learning-Based Benchmarking Framework for Lane Segmentation in the Complex and Dynamic Road Scenes
Improved Semantic Segmentation of Low-Resolution 3D Point Clouds Using Supervised Domain Adaptation
One of the key challenges in applying deep learning to solve real-life problems is the lack of large annotated datasets. Furthermore, for a deep learning model to perform well on the test set, all samples in the training and test sets should be independent and identically distributed (i.i.d.), which means that test samples should be similar to the samples that were used to train the model. In many cases, however, the underlying training and test set distributions are different. In such cases, it is common to adapt the test samples by transforming them to their equivalent counterparts in the
Improved estimation of the cardiac global function using combined long and short axis MRI images of the heart
Towards Efficient Online Topic Detection through Automated Bursty Feature Detection from Arabic Twitter Streams
Detecting trending topics or events from Twitter is an active research area. The first step in detecting such topics focuses on efficiently capturing textual features that exhibit an unusual high rate of appearance during a specific timeframe. Previous work in this area has resulted in coining the term "detecting bursty features" to refer to this step. In this paper, TFIDF, entropy, and stream chunking are adapted to investigate a new technique for detecting bursty features from an Arabic Twitter stream. Experimental results comparing bursty features extracted from Twitter streams, to Twitter
Traffisense: A smart integrated visual sensing system for traffic monitoring
Intelligent camera systems provide an effective solution for road traffic monitoring with traffic stream characteristics, such as volumes and densities, continuously computed and relayed to control stations. However, developing a functional vision-based traffic monitoring system is a complex task that entails the creation of appropriate visual sensing platforms with on-board visual analytics algorithms, integration of versatile technologies for data provision and stream management, and development of data visualization techniques suitable for end-users. This paper describes TraffiSense, a
An approach for extracting and disambiguating arabic persons' names using clustered dictionaries and scored patterns
Building a system to extract Arabic named entities is a complex task due to the ambiguity and structure of Arabic text. Previous approaches that have tackled the problem of Arabic named entity recognition relied heavily on Arabic parsers and taggers combined with a huge set of gazetteers and sometimes large training sets to solve the ambiguity problem. But while these approaches are applicable to modern standard Arabic (MSA) text, they cannot handle colloquial Arabic. With the rapid increase in online social media usage by Arabic speakers, it is important to build an Arabic named entity
Extreme Points Derived Confidence Map as a Cue for Class-Agnostic Interactive Segmentation Using Deep Neural Network
To automate the process of segmenting an anatomy of interest, we can learn a model from previously annotated data. The learning-based approach uses annotations to train a model that tries to emulate the expert labeling on a new data set. While tremendous progress has been made using such approaches, labeling of medical images remains a time-consuming and expensive task. In this paper, we evaluate the utility of extreme points in learning to segment. Specifically, we propose a novel approach to compute a confidence map from extreme points that quantitatively encodes the priors derived from
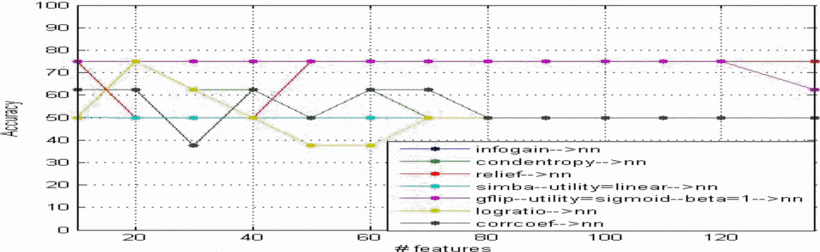
Feature selection in computer aided diagnostic system for microcalcification detection in digital mammograms
In this paper an approach is proposed to develop a computer-aided diagnosis (CAD) system that can be very helpful for radiologist in diagnosing microcalcifications' patterns in digitized mammograms earlier and faster than typical screening programs and showed the efficiency of feature selection on the CAD system. The proposed method has been implemented in four stages: (a) the region of interest (ROI) selection of 32x32 pixels size which identifies clusters of microcalcifications, (b) the feature extraction stage is based on the wavelet decomposition of locally processed image (region of
Towards scalable and cost-aware bioinformatics workflow execution in the cloud - Recent advances to the tavaxy workflow system
Cloud-based scientific workflow systems can play an important role in the development of cost effective bioinformatics analysis applications. So far, most efforts for supporting cloud computing in such workflow systems have focused on simply porting them to the cloud environment. The next due steps are to optimize these systems to exploit the advantages of the cloud computing model, basically in terms of managing resource elasticity and the associated business model. In this paper, we introduce new advancements in designing scalable and cost-effective workflows in the cloud using the Tavaxy
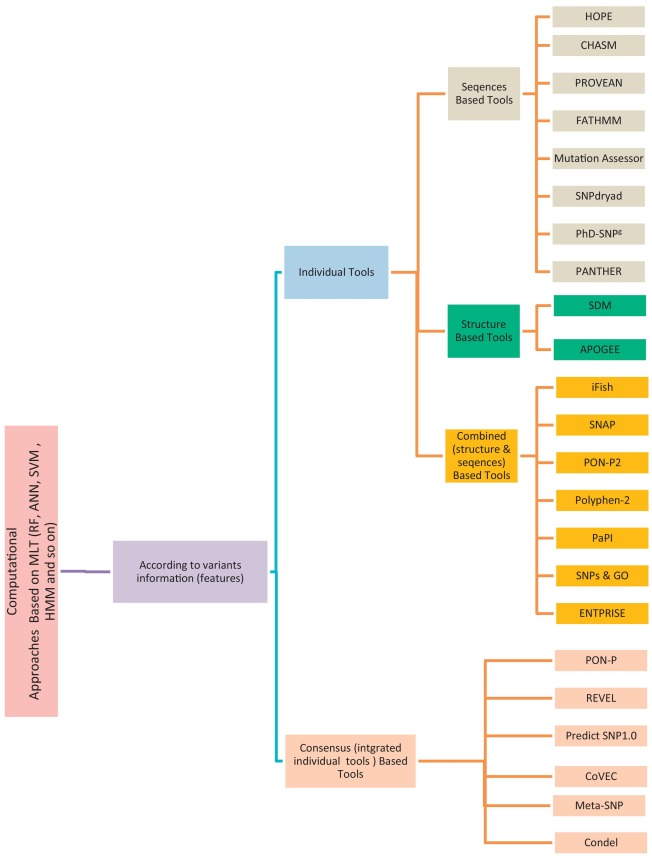
A review study: Computational techniques for expecting the impact of non-synonymous single nucleotide variants in human diseases
Non-Synonymous Single-Nucleotide Variants (nsSNVs) and mutations can create a diversity effect on proteins as changing genotype and phenotype, which interrupts its stability. The alterations in the protein stability may cause diseases like cancer. Discovering of nsSNVs and mutations can be a useful tool for diagnosing the disease at a beginning stage. Many studies introduced the various predicting singular and consensus tools that based on different Machine Learning Techniques (MLTs) using diverse datasets. Therefore, we introduce the current comprehensive review of the most popular and recent
Pagination
- Page 1
- Next page ››