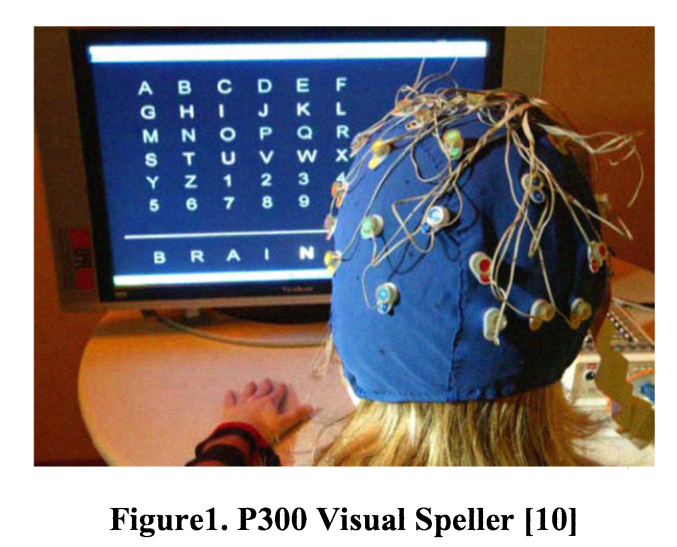
Machine learning methodologies in P300 speller brain-computer interface systems
Brain-Computer Interfaces (BCI) is a one kind of communication system that enables control of devices or communication with others only through brain signal activities without using motor activities. P300 Speller is a BCI paradigm that helps disabled subjects to spell words by means of their brain signal activities. This paper tries to demonstrate the performance of different machine learning algorithms based on classification accuracy. Performance has been evaluated on the data sets acquired using BC12000's P300 Speller Paradigm provided by BCI competitions II (2003) & III (2004) organizers for three subjects. As a primary stage, a preprocessing was applied on the samples in order to extract the most significant features before introducing them to machine learning algorithms. The algorithms applied are Bayesian Linear Discriminant Analysis (BLDA), linear Support Vector Machine (SVM), Fisher Linear Discriminant Analysis (FLDA), Generalized Anderson's Task linear classifier (GAT), Linear Discriminant Analysis (LDA). BLDA and SVM yielded the highest accuracy for all 3 subjects. Principal Component Analysis (PCA) was applied on the data presented to the BLDA classifier for dimensionality reduction. The results obtained after this stage were slightly lower than those without reduction but they are still acceptable. This advantage could help in using P300 Speller BCI system beyond mere proof-of concept systems and allow their widespread application out of laboratories.