Breadcrumb
Practical distributed computation of maximal exact matches in the cloud
Computation of maximal exact matches (MEMs) is an important problem in comparing genomic sequences. Optimal sequential algorithms for computing MEMs have been already introduced and integrated in a number of software tools. To cope with large data and exploit new computing paradigms like cloud computing, it is important to develop efficient and ready-to-use solutions running on distributed parallel architecture. In a previous work, we have introduced a distributed algorithm running on a computer cluster for computing the MEMs. In this paper, we extend this work in two directions: First, we
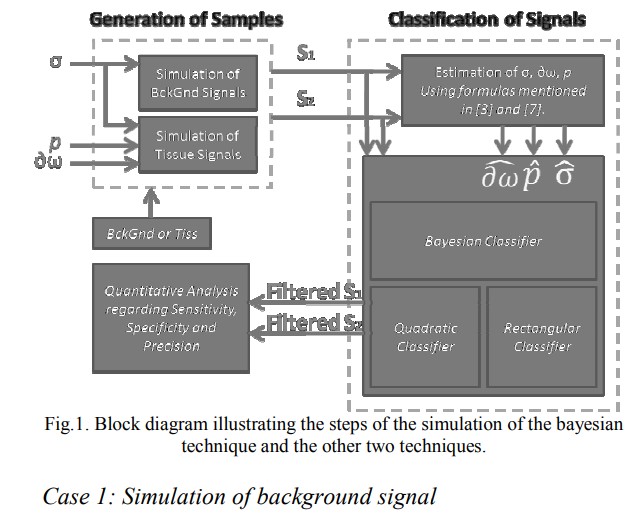
Performance evaluation of cardiac MRI image denoising techniques
Black-blood cardiac Magnetic Resonance Imaging (MRI) plays an important role in diagnosing a number of heart diseases. The technique suffers inherently from low contrast-tonoise ratio between the myocardium and the blood. In this work, we examined the performance of different classification techniques that can be used. The three techniques successfully removed the noise with different performance. Numerical simulation has been done to quantitatively evaluate the performance of each technique. © 2008 IEEE.
Emotional tone detection in Arabic tweets
Emotion detection in Arabic text is an emerging research area, but the efforts in this new field have been hindered by the very limited availability of Arabic datasets annotated with emotions. In this paper, we review work that has been carried out in the area of emotion analysis in Arabic text. We then present an Arabic tweet dataset that we have built to serve this task. The efforts and methodologies followed to collect, clean, and annotate our dataset are described and preliminary experiments carried out on this dataset for emotion detection are presented. The results of these experiments
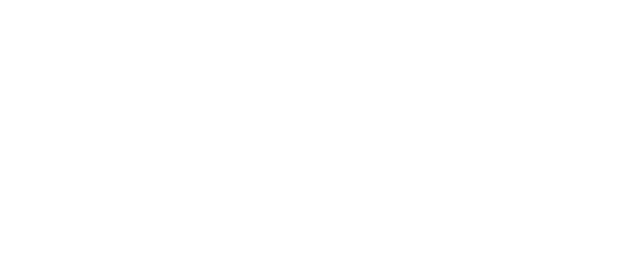
Using deep neural networks for extracting sentiment targets in arabic tweets
In this paper, we investigate the problem of recognizing entities which are targeted by text sentiment in Arabic tweets. To do so, we train a bidirectional LSTM deep neural network with conditional random fields as a classification layer on top of the network to discover the features of this specific set of entities and extract them from Arabic tweets. We’ve evaluated the network performance against a baseline method which makes use of a regular named entity recognizer and a sentiment analyzer. The deep neural network has shown a noticeable advantage in extracting sentiment target entities
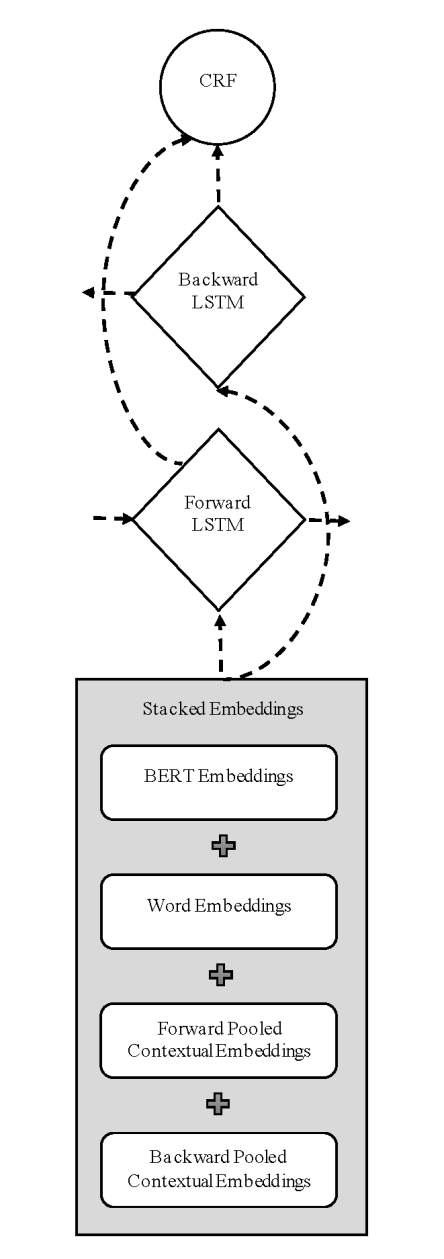
A Multi-Embeddings Approach Coupled with Deep Learning for Arabic Named Entity Recognition
Named Entity Recognition (NER) is an important task in many natural language processing applications. There are several studies that have focused on NER for the English language. However, there are some limitations when applying the current methodologies directly on the Arabic language text. Recent studies have shown the effectiveness of pooled contextual embedding representations and significant improvements in English NER tasks. This work investigates the performance of pooled contextual embeddings and bidirectional encoder representations from Transformers (BERT) model when used for NER on
MoArLex: An Arabic Sentiment Lexicon Built Through Automatic Lexicon Expansion
Research addressing Sentiment Analysis has witnessed great attention over the last decade especially after the huge increase in social media networks usage. Social networks like Facebook and Twitter generate an incredible amount of data on a daily basis, containing posts that discuss all kinds of different topics ranging from sports and products to politics and current events. Since data generated within these mediums is created by users from all over the world, it is multilingual in nature. Arabic is one of the important languages recently targeted by many sentiment analysis efforts. However
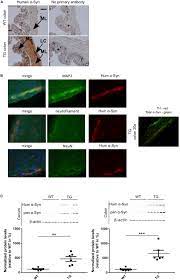
Enriched environmental conditions modify the gut microbiome composition and fecal markers of inflammation in parkinson’s disease
Recent findings suggest an implication of the gut microbiome in Parkinson’s disease (PD) patients. PD onset and progression has also been linked with various environmental factors such as physical activity, exposure to pesticides, head injury, nicotine, and dietary factors. In this study, we used a mouse model, overexpressing the complete human SNCA gene (SNCA-TG mice) modeling familial and sporadic forms of PD to study whether environmental conditions such as standard vs. enriched environment changes the gut microbiome and influences disease progression. We performed 16S rRNA DNA sequencing
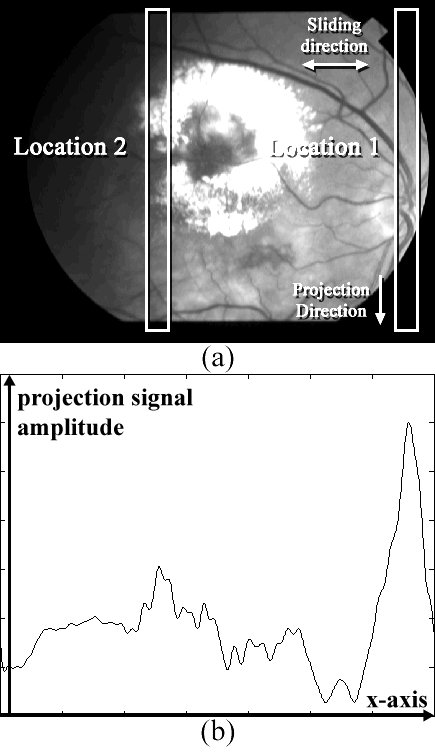
Ultrafast localization of the optic disc using dimensionality reduction of the search space
Optic Disc (OD) localization is an important pre-processing step that significantly simplifies subsequent segmentation of the OD and other retinal structures. Current OD localization techniques suffer from impractically-high computation times (few minutes/image). In this work, we present an ultrafast technique that requiresless than a second to localize the OD. The technique is based on reducing the dimensionality of the search space by projecting the 2D image feature space onto two orthogonal (x- and y-) axes. This results in two 1D signals that can be used to determine the x- and y-
EGEPT: Monitoring middle east genomic data
EGEPT (Middle East GenBank Post) is a database that monitors submissions to the GenBank nucleotide database from Middle East countries. The data in EGEPT is browsable by country, institute, author, organism, and related publications. Statistics about the dataset is provided and charts that compare the Middle East countries to each other are automatically generated. EGEPT revealed that Qatar, Egypt, Oman, Tunisia, and Morocco are leading in terms of sequence submissions and related publications. However, the total submissions of all Arab countries is greatly lagging behind other Middle East
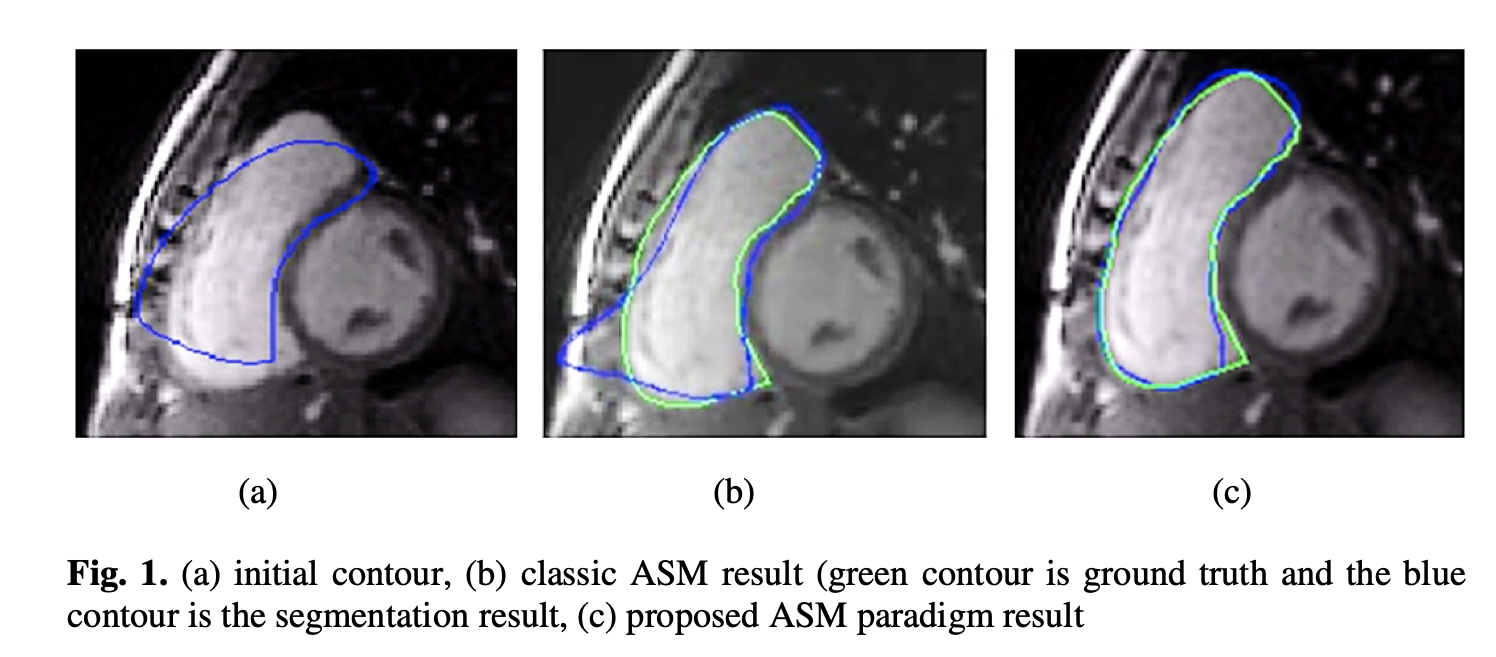
Active shape model with inter-profile modeling paradigm for cardiac right ventricle segmentation
In this work, a novel active shape model (ASM) paradigm is proposed to segment the right ventricle (RV) in cardiac magnetic resonance image sequences. The proposed paradigm includes modifications to two fundamental steps in the ASM algorithm. The first modification includes employing the 2D-Principal Component Analysis (PCA) to capture the inter-profile relations among shape’s neighboring landmarks and then model the inter-profile variations between the training set. The second modification is based on using a multi-stage searching algorithm to find the best profile match based on the best
Pagination
- Previous page ‹‹
- Page 11
- Next page ››